The beauty and healthcare industries’ understanding of skin conditions, the personalization of treatments, and the creation of new products have all been transformed by the significant role that machine learning (ML) plays in the analysis of skincare data. Here’s a breakdown of its impact:
- Personalized Skincare Solutions
Skin Type Classification: ML algorithms analyze images and customer data to classify skin types (e.g., oily, dry, combination) and conditions (e.g., acne, eczema, wrinkles).
Customized Recommendations: By analyzing a user’s skin profile, genetics, environment, and lifestyle, ML models recommend personalized skincare routines and products. - Image Analysis and Skin Condition Detection
Computer Vision: To identify skin conditions like acne, rosacea, and pigmentation from images, ML models, particularly deep learning techniques like convolutional neural networks (CNNs), are used. Early Detection: By analyzing dermatoscopic images with high accuracy, ML aids in the early identification of serious skin conditions like melanoma. - Consumer Behavior Analysis
Sentiment Analysis: ML analyzes product reviews and social media data to understand consumer satisfaction and emerging skincare trends.
Preference Prediction: Models predict future purchasing behavior by learning from past customer data, enabling targeted marketing. - Formulation Development
Ingredient Optimization: ML helps identify effective ingredient combinations by analyzing vast datasets on how different compounds affect various skin conditions.
Reducing Trial Time: Algorithms simulate skin reactions to new formulations, reducing the time and cost of physical testing.
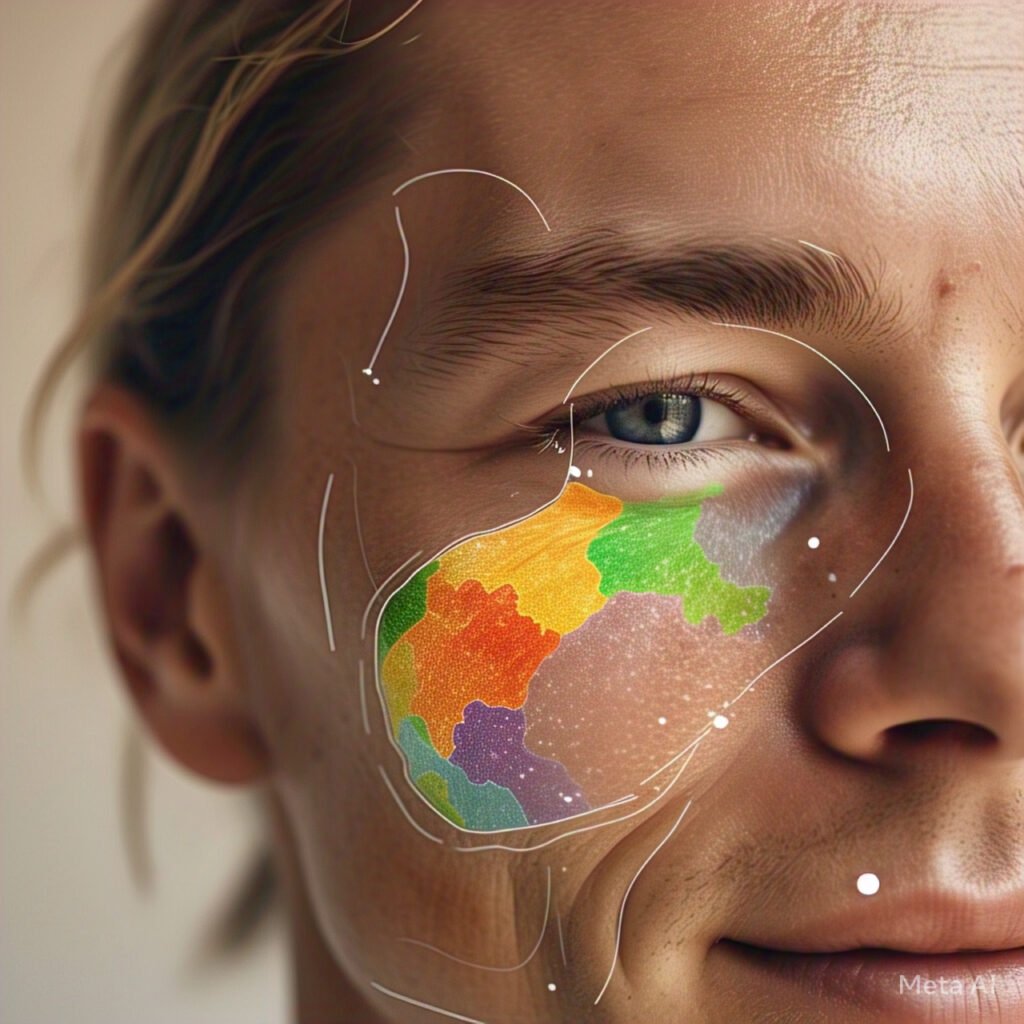
- Monitoring Skin Changes Over Time
Progress Tracking: ML can track a user’s skin condition over time through regular image analysis, providing feedback on product efficacy.
Predictive Analytics: ML makes predictions about how external factors affect skin health by modeling environmental factors like pollution and UV exposure. 6. Genomic and Microbiome Analysis
DNA-based Skincare: ML analyzes genetic data to offer skincare solutions tailored to an individual’s genetic predispositions.
Microbiome Insights: Machine learning examines skin microbiome data to suggest probiotics or other treatments for balanced skin health. - Teledermatology and Remote Diagnostics
Remote Assessments: ML-powered platforms allow users to upload skin images for instant analysis and preliminary diagnosis.
Accessibility: These tools provide skincare insights to people in remote areas who lack direct access to dermatologists.
Challenges and Future Directions:
Data Privacy: Handling sensitive personal and medical data requires stringent privacy protocols.
Bias and Fairness: ML models must be trained on diverse datasets to avoid biased recommendations across skin tones and types.
Explainability: Transparent algorithms are crucial to ensuring consumer trust and medical compliance.
Would you like to explore a specific aspect in more detail?
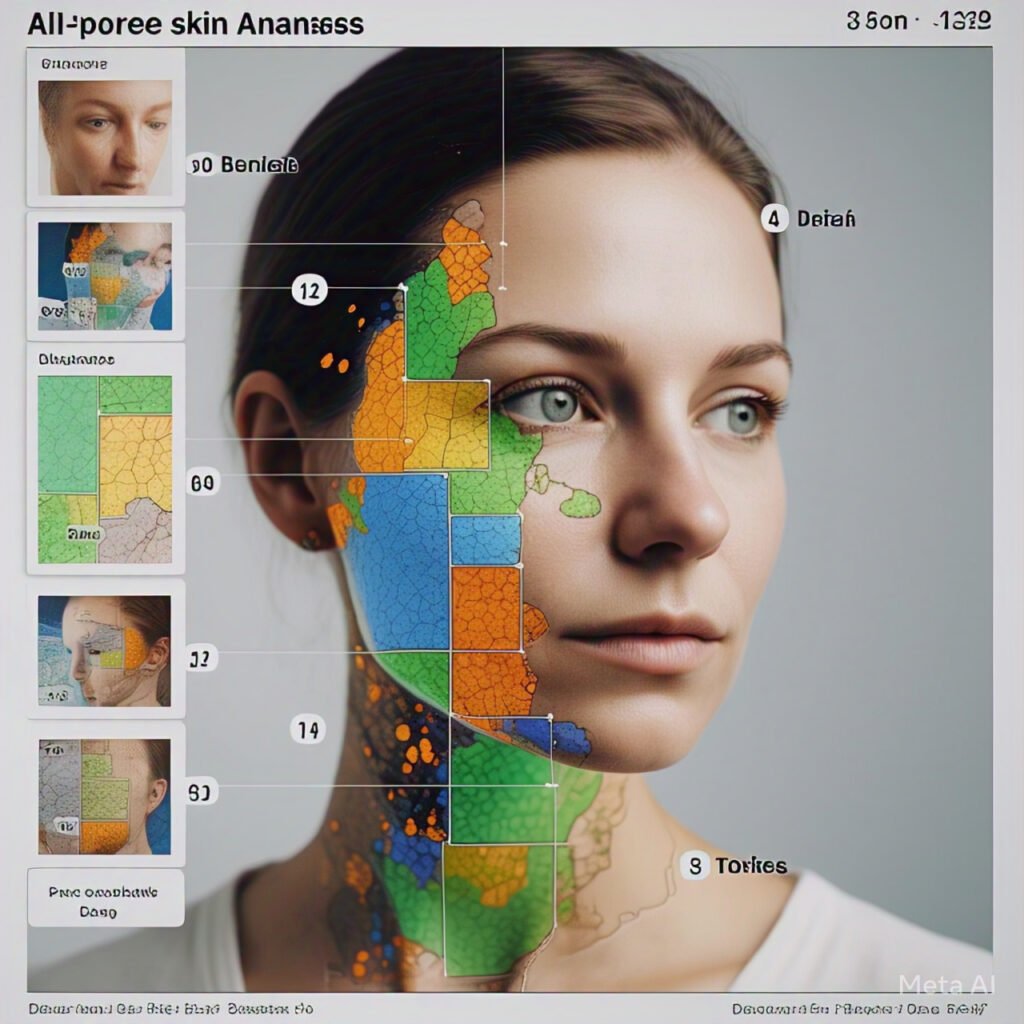